各式各样的 Attention
# 1. self-attention 的问题
# 1.1 复习一下 self-attention
假设 sequence length = N,那么一次计算中会有 N 个 key,N 个 query,两两做 dot-product 产生一个 N * N 的 Attention Matrix:
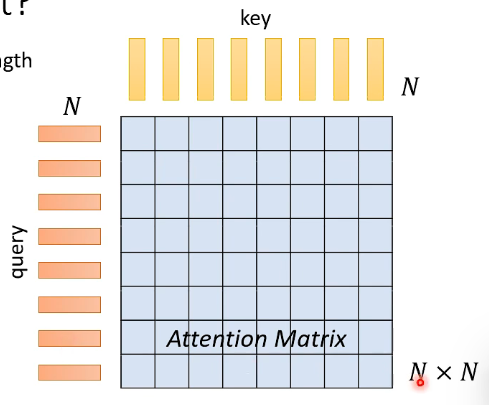
拿到 Attention Matrix 后,对 value vector 做 weight sum 就可以了。
而 self-attention 的痛点在于:计算 N * N 的 Attention Matrix 的计算量可能非常惊人,尤其是 sequence length N 非常长时。
# 1.2 Notice
- Self-attention is only a module in a larger network.
- Self-attention dominates computation when N is large in Transformer. 所以当 N 不是很大时,可能整个 network 受 feed forward 等其他 module 的 dominate,此时改进 self-attention 的效果也许对加快整体的训练不会有太大改进。
- Usually developed for image processing. 因为假如要处理一个 256 * 256 的 image,那此时 N = 256 * 256,计算量将非常大。
下面就要讲对 Self-Attention 的改进了。
# 2. Skip Some Calculations with Human Knowledge
idea:在计算 Attention Matrix 时,也许我们不需要计算每一个值,也许我们可以凭借 human knowledge 来预先填上一些值。
# 2.1 Local Attention / Truncated Attention
一种想法是,有些情况在做 Attention 的时候,有些位置不需要看整个 sequence,而是只需要看左右邻居就可以理解一个位置的 token 存有什么样的资讯:
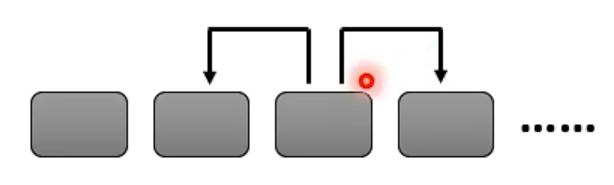
这样的话,我们可以直接把更长距离的 attention weight 设为 0,即:
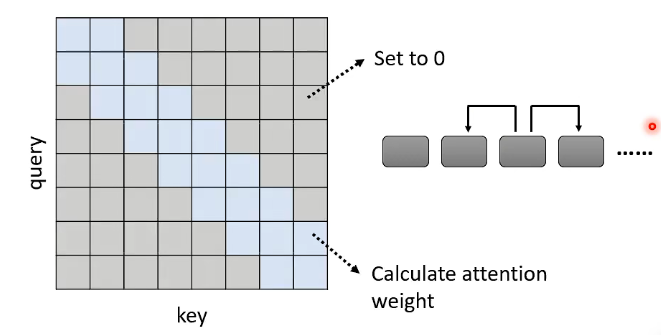
- 灰色部分直接被设为 0
- 蓝色部分才需要去计算 attention weight
上面这种方法称为 Local Attention / Truncated Attention。但这存在一个问题:每次做 attention 时只能看得到周围一小个范围的资讯,那这样的话就会跟 CNN 很像了。这种方式会加快你的运算,但不一定会带来好的结果。
# 2.2 Stride Attention
既然说只看邻居不好,那我们就看一下远一点的邻居:

- 在这里,我们先跳两格,看三格位置处的资讯,这样就可以看到更大的范围的资讯,把 Attention Matrix 网格画出来的话就是这样(灰色设为 0,青色才需要计算):
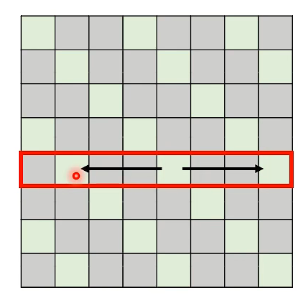
这种方式称为 Stride Attention。上面例子是空两格,当然也可以空一格、空三格 ….
# 2.3 Global Attention
刚刚所说的是只能知道周围发生了什么事,但如果想知道一整个 sequence 发生了什么事情,就需要用 Global Attention。
这需要在原来的 sequence 上加一些 special token,这些 special token 代表这些 position 需要做 Global Attention。
Global Attention 会做两件事:
- Attend to every token.(也就是从 sequence 的每一个 token 里面收集资讯) --> collect global information.
- Attended by every token. (也就是所有 token 都去看一下这个 special token 发生了什么资讯) --> it knows global information.
Global Attention 有两种做法:
- 直接在 original sequence 中 assign 一些 token 作为 special token。比如将作为开头 token 的 [CLS] 作为 special token,或将句号作为 special token。【如下左图】
- 外加一些额外的 token 作为 special token,这样不管 original sequence 是什么,都硬插几个 token 作为 special token。【如下右图】
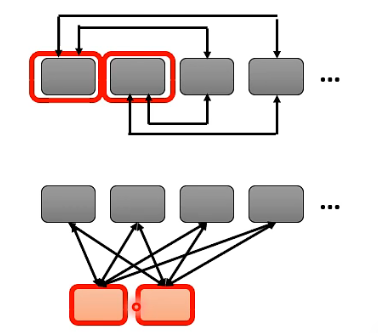
如果将 Attention Matrix 画出来的话,就会是下图这样:
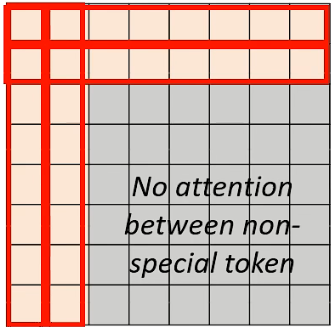
- 每一个 row 代表一个 query,每一个 column 代表一个 key
- 会发现前两个 row 都是有值的,也就是都是要做 attention 的,即前两个位置代表 special token,他们的 query 要被 attend 到所有其他人的 key 上;
- 前两个 column 也都是有值的,代表说除了 special token 以外的那些 token 在做 attention 的时候,他们的 query 都会被 attend 到前两个位置的 token 上;
- 除了前两个 token 会 attend 到其他所有人,也会被其他所有人 attend 以外,其他的 token 之间就彼此就没有往来了。
# 2.4 选择哪一种 self-attention?
上面讲了多种 self-attention 的改进方式,那选择哪一种最好呢?
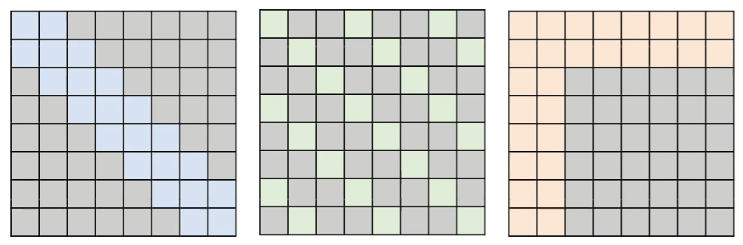
小孩子才做选择… Different heads use different patterns. 比如一些经典模型:
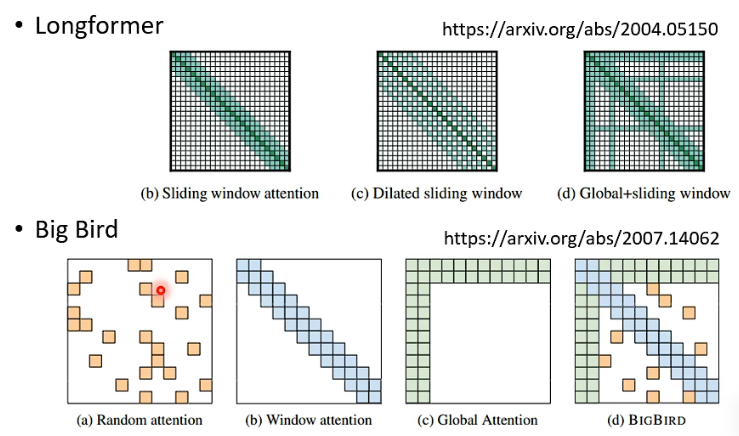
# 3. Can we only focus on Critical Parts?
之前我们讲的是人工的方式告诉你哪些部分的 attention 也许不用计算了,但这种方式并不一定是最好的,因此想能不能变成以 data driven 的方式来寻找哪些不用计算了。
一个 Attention Matrix 可能如下所示:
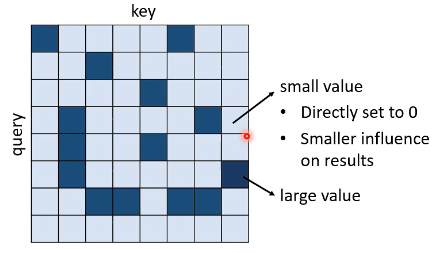
会有一部分的 attention weight 是很小的,如果把这些 small value 直接设为 0,对结果的影响也是很小的,却能加快计算。因此现在的问题是:How to quickly estimate the portion with small attention weights?
# 3.1 Clustering
这一技术在 Reformer (opens new window) 和 Routing Transformer (opens new window) 都有运用。
# Step 1
对 query vector 和 key vector 基于 similarity 做 clustering,分成几种不同类型的 vector。下图示例是分成了 4 种 vector:
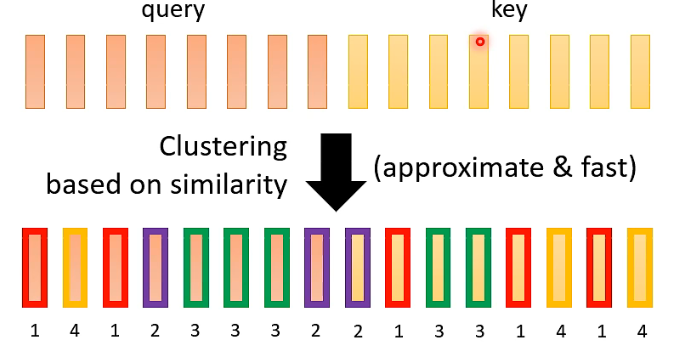
- 上图中,相同颜色边框的 vector 是同一类的 vector。
也许你会怀疑做 clustering 本身的计算量会不会有影响。如果 clustering 的计算复杂度达到了 sequence n 的
# Step 2
相同 cluster 之间的 vector 计算 attention weight,其余的直接补 0:
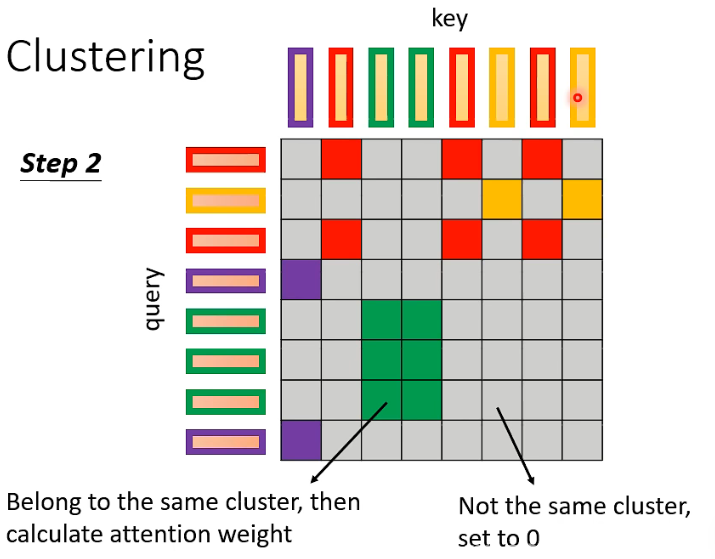
到目前为止,所讲的方法还都是基于人类对这个问题的理解来决定要不要计算 attention weight,那有没有办法把“要不要计算 attention”这件事情用 learn 的方式直接把它学出来呢?这是有可能的。
# 3.2 Learnable Pattern - Sinkhorn Sorting Network
Sinkhorn Sorting Network 要做的就是“哪些地方要不要计算 attention 是直接通过 learn 的方式来得到“。
首先需要产生一个由 0/1 组成的 Matrix,值为 1 的位置才需要去计算 attention,如下图中深色部分代表 1:
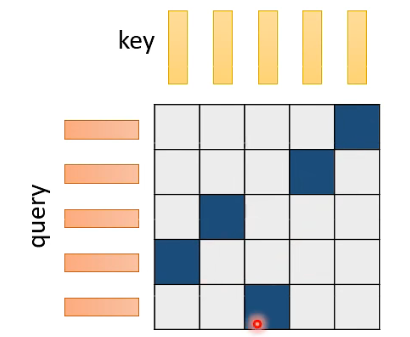
而这个 Matrix 怎么来的呢?而是需要 learn 另一个 network。input sequence 的每一个 vector 通过一个 neural network 产生另一个 vector,这个新产生的 vector 的长度需要与 sequence 一样,这样产生的一排 vector 就是
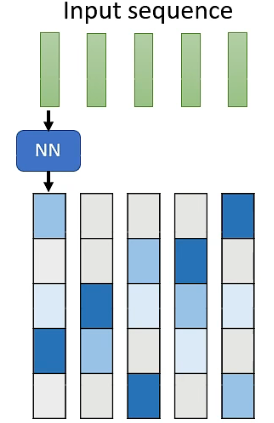
但这个新产生的一排 vector 构成的 matrix 的值是 continues 的,而我们一开始想要的 matrix 是 binary 的,所以我们需要做的就是把这个 continues 的 matrix 转成 binary 的 matrix,而这一步也是这个 model 的核心工作,具体怎么运作可以参考相关的文献 (opens new window)。
# 3.3 Do we need full attention matrix?
Linformer (opens new window) 的作者发现,完整的 attention martrix 大部分都是 low rank 的,从这个角度讲就是它包含很多 redundant columns,所以我们根本就不需要一个
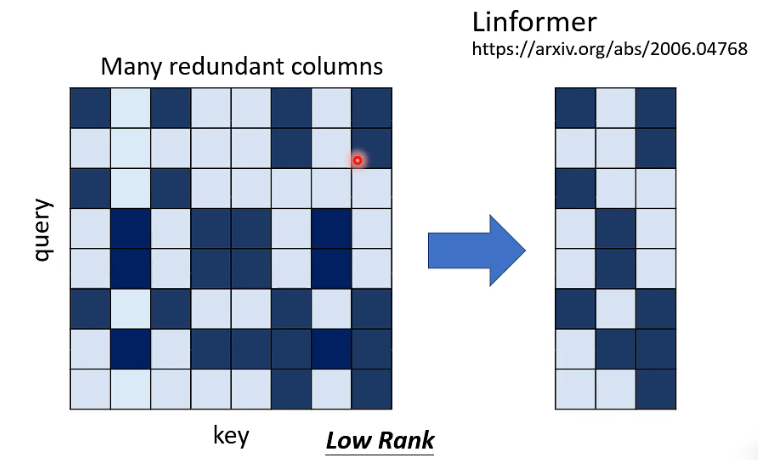
实际上的做法就是从 N 个 key 里面选出最具代表性的 K 个 key 用于计算 attention matrix,这样就不需要算一个完整的 attention matrix 了:
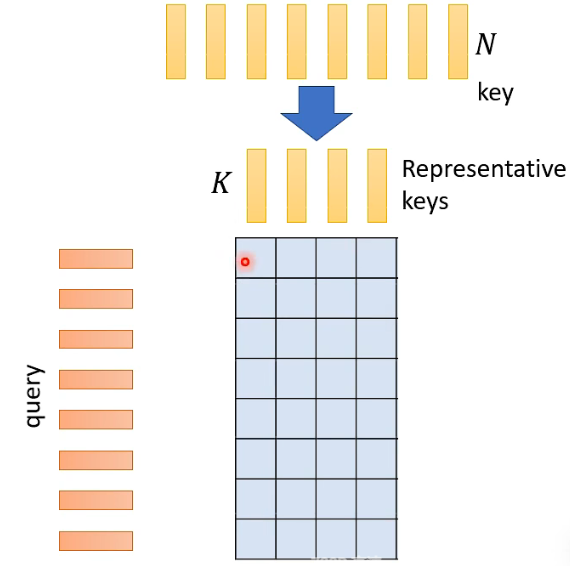
那怎样用这个 attention matrix 来产生 Attention Layer 的 output 呢?选出的 K 个 key 也具有与之对应的 K 个 value,然后就可以每 K 个 attention weights 与 K 的 value 做 weighted sum 得到一个 output vector:
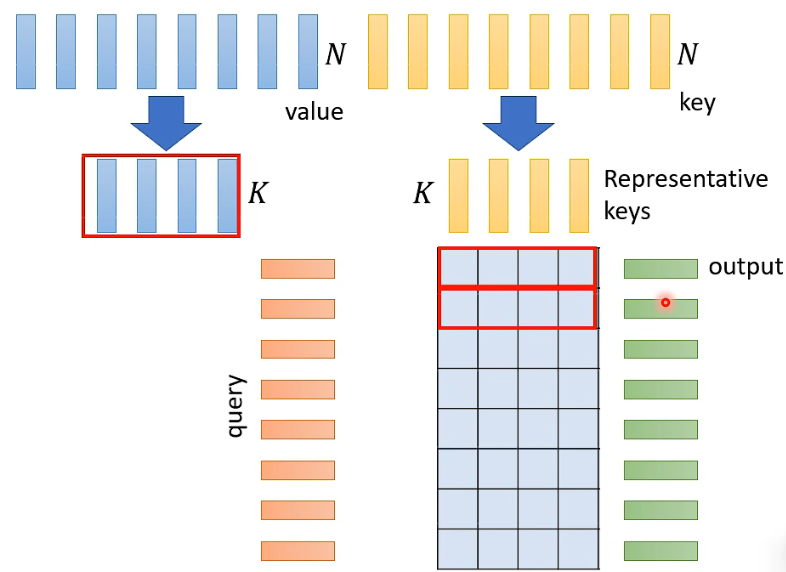
到这里,你也许有个疑问:为什么是选 K 个有代表性的 key,而不是 query 呢?我们可以减少 query 的数量吗?我们注意,output 的 length 是与 query 的数量一致的,如果 query 减少的话,ouput sequence 也会相应减小。这种减小会有影响吗?这要看具体任务,比如 test classification,看完一遍 sequence 只需产生一个 label,那这时真的可以让 query 也变少,但假如具体任务是每一个 sequence 的位置都需要产生一个 label,那这时就有问题了,也就不能减少 query 的数量了。
下面讲怎么选有代表性的 key?一篇叫做 Compressed Attention 的 paper 的做法是用 CNN 来扫过 input sequence 从而使其变短,从而作为有代表性的 key。另外一个 Linformer 是把 N 个 key 拼成一个
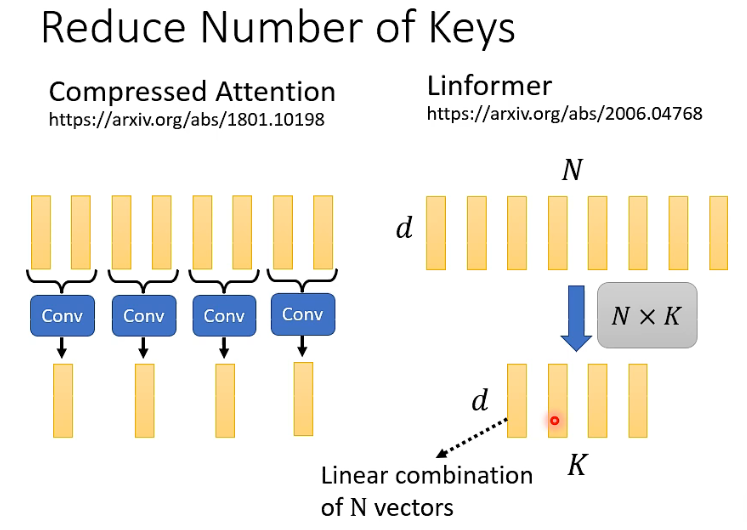
- Linformer 中我们可以看到,其实选出来的每一个有代表的 key 都是以前 N 个 key vector 的 linear combination。
# 4. 从矩阵运算的角度考虑简化
其实 Attention Mechianism 就是 three-matrix multiplication,我们先来复习一下这个运算过程:
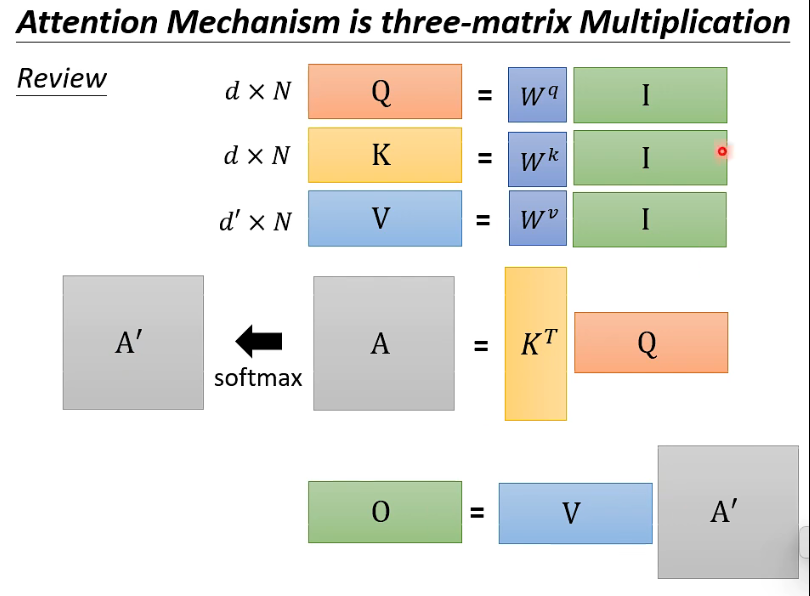
- 注意这里 V 矩阵的 size
是可以与 Q、K 的不一样的,大部分情况下我们是让 ,但其实也是可以不相等的。
现在我们先做一个假设,也就是没有从
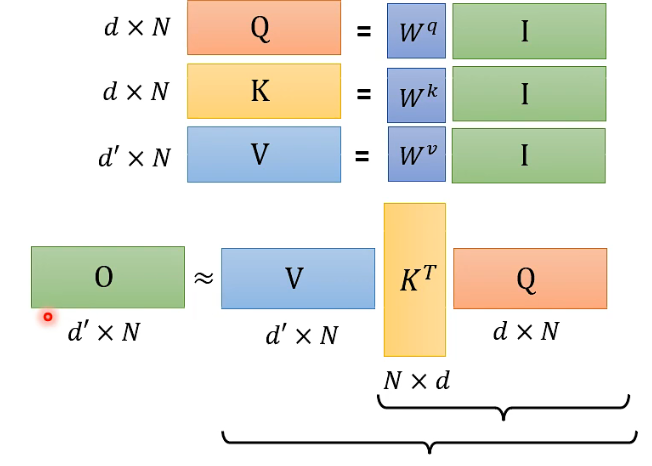
这个过程其实是可以加速的:
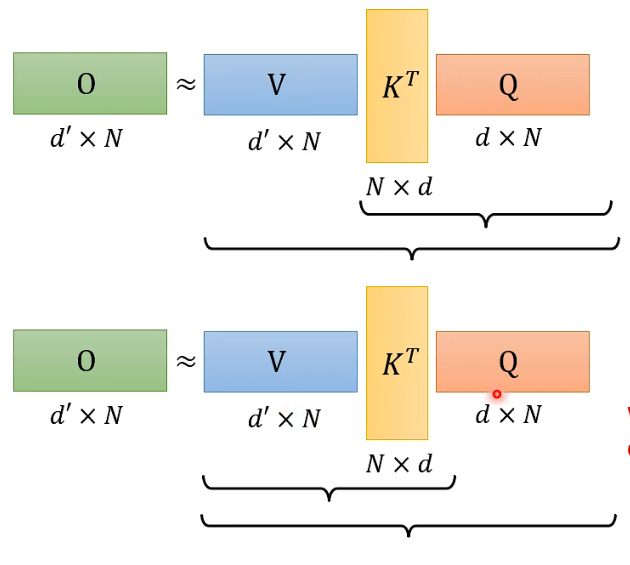
在上图中,上下两种结合方式的计算结果
刚刚是假设先忽略掉 softmax 过程,那把 softmax 拿回来会不会有影响呢?// TODO:数学很复杂,可以看李宏毅原视频,这里也涉及到多篇 paper。
# 5. Do we need q and k to compute attention?
做 self-attention,就一定需要用 q 和 k 去计算出 attention weights 吗?不一定,可以参考一种做法:Synthesizer!
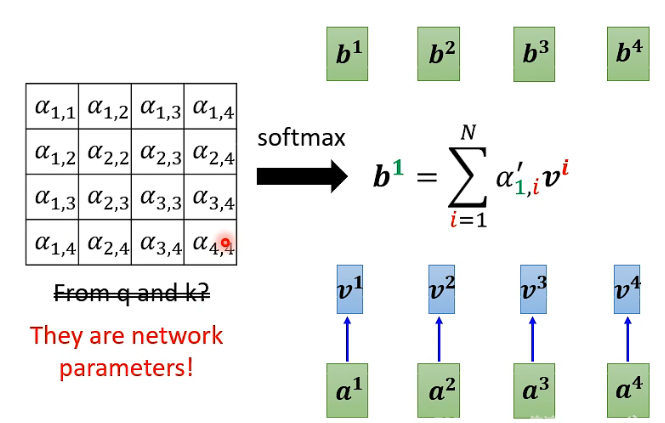
这里的 Attention Matrix 里有
但有人可能会问,这样做的话对于不同的 input sequence,它们的 attention weights 都成一样的了,这样 performance 会变差吗?不会!就这样。所以这个 Synthesizer 让我们重新思考到底 attention 的价值到底是什么。
更进一步,人们开始思考处理 sequence 一定要用 attention 吗,于是开始寻找有没有可能丢掉 attention,去寻找 attention-free 的方法,所以有一系列的 paper 直接用 MLP 来处理 sequence:
- Fnet: Mixing tokens with fourier transforms (opens new window)
- Pay Attention to MLPs (opens new window)
- MLP-Mixer: An all-MLP Architecture for Vision (opens new window)
# 6. Summary
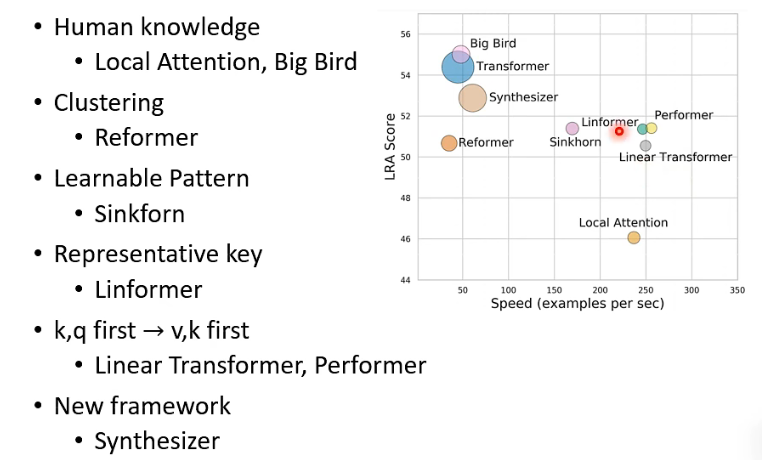
- 上面的坐标图是在一个 self-attention benchmark 上的结果,越往右表示速度越快,越往上表示 score 越好,即 performance 越好,圆圈大小代表运算所用 memory 大小,越大的圆圈代表所用越大的 memory。